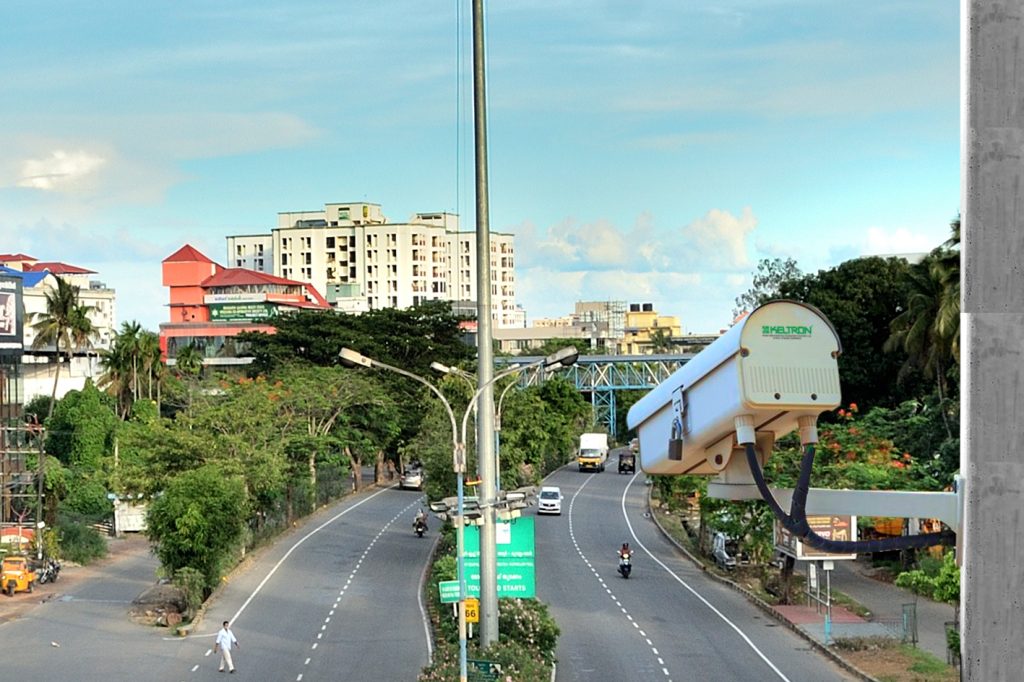
The topic of AI traffic cameras has become the talk of Kerala, with everyone discussing the latest technology that is revolutionising the way we manage traffic on our roads and highways. But what exactly is an AI camera, and what makes it such a fascinating and relevant topic of discussion?
Can a camera analyse an image or footage?
Yes! AI cameras can.
How? An AI camera is just an electronic device. Then how does it identify and report traffic offenses?
However, it’s important to note that this is not a magic device and relies on underlying technology. This blog explores AI traffic cameras, their advanced technology, and their impact on traffic management and road safety. Before discussing this, I hope that everyone knows what artificial intelligence is. AI is when machines think like humans, using algorithms and data analysis to solve problems and learn from experience. It’s like giving machines the ability to be really smart and do things that humans can do.
How does a machine get this ability? Is it possible to learn this intelligence by the machine itself? No! Simply put, we humans make them intelligent by giving them training with huge amounts of data and algorithms. We call this ‘machine learning’. They use special programmes to get smarter and solve problems without needing someone to tell them what to do.
How Does an AI Traffic Camera Work?
The “Fully Automated Traffic Enforcement System,” according to the traffic department, uses AI cameras to catch and notify vehicle owners about traffic violations. These cameras are powered by solar energy and send data to the control room using 4G LTE technology. In the first step, the cameras scan every vehicle and send the information to the control room. The control room receives photos of the vehicles and drivers that have broken traffic laws. Within 24 hours of a violation, the vehicle owner is informed about it through courier, email, and mobile phone messages.
Let’s discuss it in detail.
What offenses can an AI traffic camera report?
Initially, the AI cameras in Kerala will spot four types of violations. Not wearing a helmet or seat belt, carrying more than two people on a two-wheeler, using phones while driving, and running red lights. How does an AI traffic camera determine whether a violation has occurred based on visuals or images? I can explain the technologies and algorithms used for this.
Image Capturing
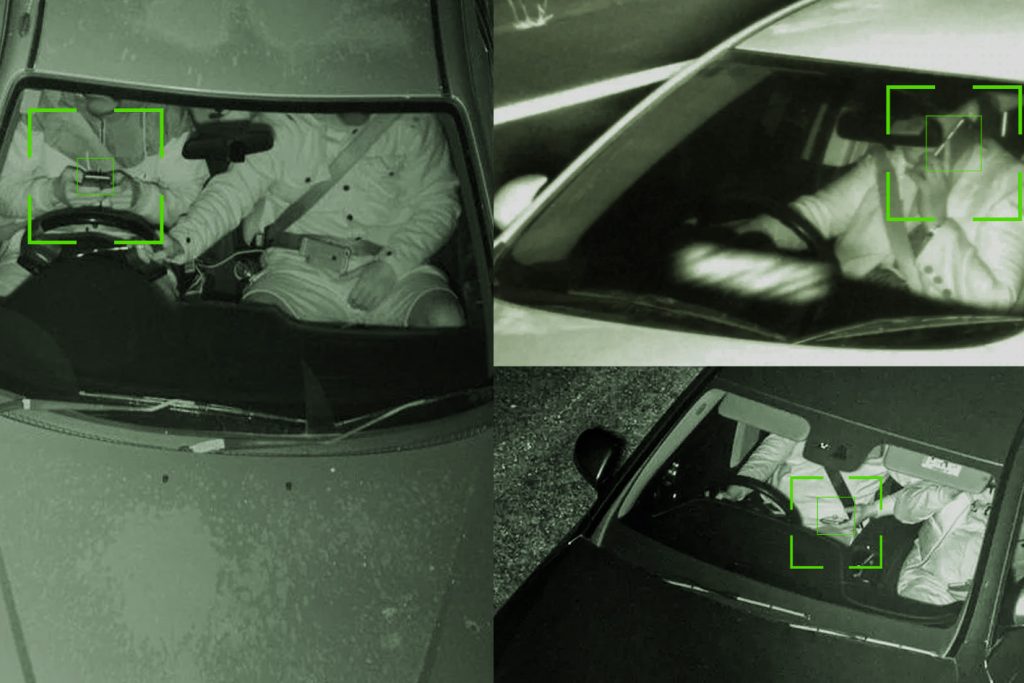
The solar-powered AI traffic cameras capture clear and high-quality images. It uses sunlight to work and captures images for surveillance purposes. These cameras are capable of operating 24 hours a day and can capture images even in low-light or nighttime conditions.
To achieve uninterrupted operation, the camera requires efficient components and optimised software that minimise energy consumption. It also needs high-efficiency solar panels that can generate enough energy from available sunlight and withstand harsh weather conditions. Adequate battery storage is essential to store energy for times when sunlight is not available.
Visual Processing System
An internal visual processing system is embedded in each AI camera. When the camera captures a visual, it analyses the image internally without sending any information to a remote server. Only if a violation is detected, the camera send the necessary data to the server, enabling it to report the offence independently. Here, we have to know about edge computing.
What is the main purpose of edge computing here?
AI traffic cameras process image data locally with edge computing, eliminating the need for distant server transmission. This helps the AI camera quickly analyse the image and identify any possible violations without any delays. It reduces the amount of data sent over the network, making real-time tasks like traffic monitoring faster and more efficient.
How can it identify offences from a huge amount of visuals? Or how will it decide if it’s an offence or not?
Here, we have to understand computer vision. Computer vision algorithms in an AI traffic camera analyse visual data to identify patterns, objects, and colours. They can track object movement, detect traffic violations, spot potential threats, and recognise specific behaviours or actions.
Rule-Based Violation Detection
Rules have been programmed into the AI cameras. Also, many sensors are attached to help identify red line violations, mobile phone use while driving, etc. To detect violations of these rules, many AI-trained models have been running on them. The models learn to identify objects and features in images by analysing labeled data and recognising patterns. This allows the cameras to accurately identify rule violations based on the learned patterns and features in real time.
This is the time to understand data labeling, the relationship between ML models and data labeling, data labeling services and their providers, etc. Let’s go through all of this.
Trained Model: Data Labeling
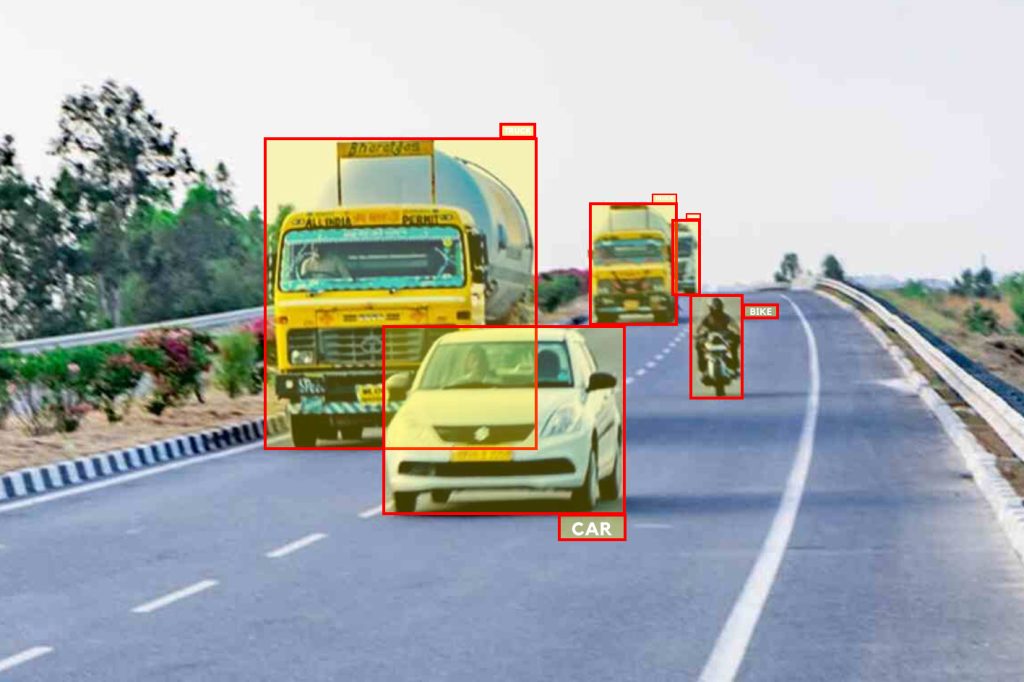
Data labeling is the process of assigning meaningful and relevant labels to data points used to train AI models. The labeled data provides the foundation for the model to learn and make predictions based on patterns identified in the data. Using specialized tools and platforms is essential to ensuring accuracy and quality in the labeling process.
Data labeling is a basic and important part of training AI models. The system works properly and runs successfully only if the basic level is error-free. This means data labeling should be well qualified and finished. Who will label this huge amount of data? In India, over the past seven years, a company named Infolks has been progressively working to meet the rising demand for image annotation and other data labeling services.
Types of Data and Technologies Used for Labeling
To improve any AI model, the initial step involves selecting a training algorithm and validating the model using top-notch training data. Infolks helps to process the perfect training data once the type of data labeling service is selected. Providing data labeling for every kind of data such as images, text, video, audio, and 3D PowerPoint.
In traffic AI cameras, bounding box labeling is used for object detection and tracking, helping the model identify the location and size of vehicles, pedestrians, and bicycles, track their movements, and provide real-time insights to improve safety and efficiency on the road.
The contour labeling tool is used to mark important features in images or videos. In the case of AI traffic cameras, it helps identify specific details like licence plates or road signs. By using this tool to label data accurately, the AI algorithms used in AI traffic cameras can be trained to better detect and report traffic violations.
The keypoint tool in AI traffic cameras is used to identify and track specific points of interest, such as traffic signs, traffic lights, or pedestrians. This technique helps the AI model accurately identify and classify objects in the camera’s field of view, improving traffic management and safety.
Data labelers, approvers, and QC managers make up Infolks’s triple-layer quality control system. Infolks can guarantee the highest quality in its work by putting this triple-level quality assurance system into place. With accurate and high-quality output, Infolks may maintain the best output. Therefore, it’s critical for Infolks to provide accurate data.
In the future, to detect other violations, we can update it by adding a trained model to the system.
Let’s check out some algorithms working on AI traffic cameras.
Object Detection Algorithm
How does it identify a vehicle with its model, colour, size, speed, etc.? Here, we have to know about deep learning. Before discussing this with an example, I would like to mention what deep learning is.
Deep learning is a type of AI that learns from data by creating a complex network of layers that recognize patterns and make predictions. It’s like a computerized brain that can recognize things and make decisions based on what it’s learned from lots of examples. YOLO is one of the most effective and efficient algorithms for object detection used in traffic AI cameras.
Let’s check this out with an example.
For seat belt detection, a deep learning model can be trained on a dataset of labeled images of drivers wearing or not wearing seat belts. The model can be designed to use a convolutional neural network (CNN) architecture, which can learn to detect features specific to seat belts, such as their shape and texture.
Once trained, the deep learning model can be deployed on an AI traffic camera to detect whether or not a driver is wearing a seat belt in real time. The camera captures an image or video of the driver, which is then processed by the deep learning model to determine if a seat belt is being worn.
By continually training and updating the deep learning algorithm with new data, such as different vehicle types or driving behaviours, the AI camera can improve its accuracy and performance over time, making it more effective at detecting and responding to potential safety risks for vehicles on the road.
License Plate Recognition Algorithm
How does it identify the number plate and send messages to the owner’s mobile number? Here is the answer!
Identifying an image’s edges by using edge detection
Identifying the licence plate area from it.
Recognize each character by using character segmentation.
Convert this to an actual character by using OCR (optical character recognition).
By using edge detection, the LPR algorithm can isolate the licence plate from the rest of the image, reducing the chance of false readings and increasing the accuracy of the system.
Speed Recognition Algorithm
Speed recognition of traffic AI cameras capture real-time video footage of vehicles passing through the camera’s coverage area. The camera then uses computer vision and machine learning algorithms to analyze the footage and detect the speed of each vehicle. The speed recognition process involves several steps, including object detection, tracking, and speed calculation. The speed of each vehicle is calculated based on its position and movement over a period of time using complex mathematical calculations.
AI cameras equipped with solar power and wireless technology can be easily relocated from one location to another, allowing for more flexibility in their usage and monitoring capabilities. Future upgrades to these cameras could include the ability to spot one-way violations, lane straddling (driving in the middle of the queue rather than staying in one lane), and lane discipline violations.
Answer Some Questions from the Public.
How many images can an AI traffic camera capture? For how long can they store the captured images?
It can store any number of images for as long as required. It is possible to perform a backup at any given time.
Can an AI camera detect a seatbelt when a person is wearing black clothing or when a woman is wearing a shawl that partially covers her body?
No matter what clothes you wear, it can detect a seatbelt. The camera captures multiple exposures of the same person. so it can identify whether the person is wearing a seatbelt.
Will AI cameras have any exceptional cases in their rule-based algorithm?
Emergent vehicles, which need to be reached quickly, have been excluded. Fire engines, ambulances, police, and other vehicles with beacon lights are exempt. No other concessions exist.
Let’s conclude that AI traffic cameras represent a significant advancement in the field of traffic management and safety, and they are likely to become even more sophisticated and widespread in the years to come. While their impact on society is complex and multifaceted, there is no doubt that they will continue to play a vital role in ensuring safe and efficient transportation on our roads. Keep in mind that this is for our safety, not to generate money for the government.
Stay safe on the road.
Have a pleasant journey.
Remember to care for others as well.