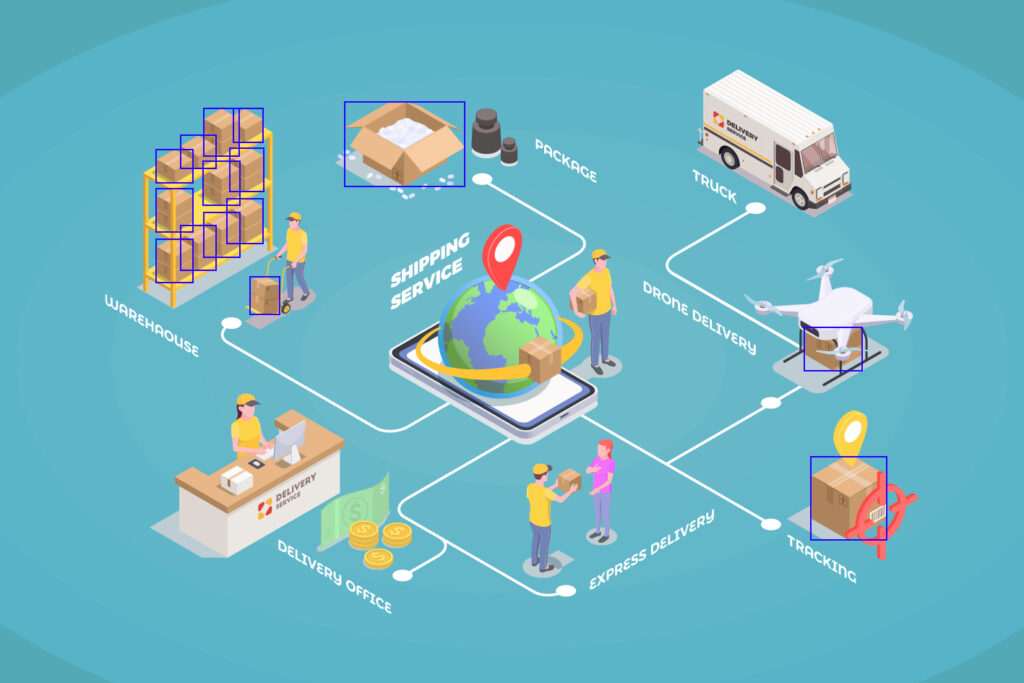
The logistics industry is undergoing a rapid transformation, powered by the integration of artificial intelligence to meet the challenges of modern supply chains. AI is reshaping logistics by streamlining routes, automating operations, improving decision-making, and advancing sustainability. Data labeling is at the core of this innovation, a critical step in preparing high-quality datasets to train AI models. Accurate labelings enable AI to make smart predictions, streamline operations, and solve complex logistics challenges for sustainable supply chains. For a deeper understanding of this topic, read our informative blog.
AI is Redefining Logistics
AI fundamentally reshapes the logistics industry, transforming traditional supply chains into smart, data-driven networks. Logistics companies leverage advanced AI to increase efficiency, reduce costs, and improve customer satisfaction. AI algorithms process vast amounts of data in real time, optimizing everything from route planning and inventory management to demand forecasting. This allows businesses to make more accurate, data-backed decisions, streamline operations, and reduce delays. AI is also driving the rise of autonomous vehicles and drones, which help speed up delivery times while reducing the need for human labor. Moreover, AI-powered systems enable more personalized services, enhance last-mile delivery accuracy, and provide predictive insights to prevent disruptions before they occur. As AI advances, it will further transform logistics, making supply chains faster, more reliable, and capable of meeting the demands of a rapidly changing market.
AI is transforming logistics through different methods like optimizing routes, improving demand forecasting, and automating warehousing. These advancements help businesses reduce waste, enhance efficiency, and streamline operations. From precise inventory management to AI-driven robots in warehouses, AI is paving the way for smarter, cost-effective logistics solutions.
Data Labeling: The Backbone of Logistics AI
Data labeling is crucial to the success of AI in logistics, as it provides the accurate, structured datasets needed for machine learning models to function effectively. By labeling data such as images of packages, roads, and vehicles, AI systems can recognize patterns and optimize tasks like route planning, demand forecasting, inventory management, and predictive maintenance. Additionally, data labeling supports the operation of autonomous vehicles, drones, and robots, enabling them to navigate efficiently and safely. AI breakthroughs in logistics rely on quality labeled data to identify trends in delivery, customer behavior, and inefficiencies. Properly labeled data ensures accurate predictions and minimizes error margins. It enhances machine learning models to optimize logistics processes, from inventory counting to real-time tracking. As AI evolves, operations in the logistics industry become more efficient and reliable.
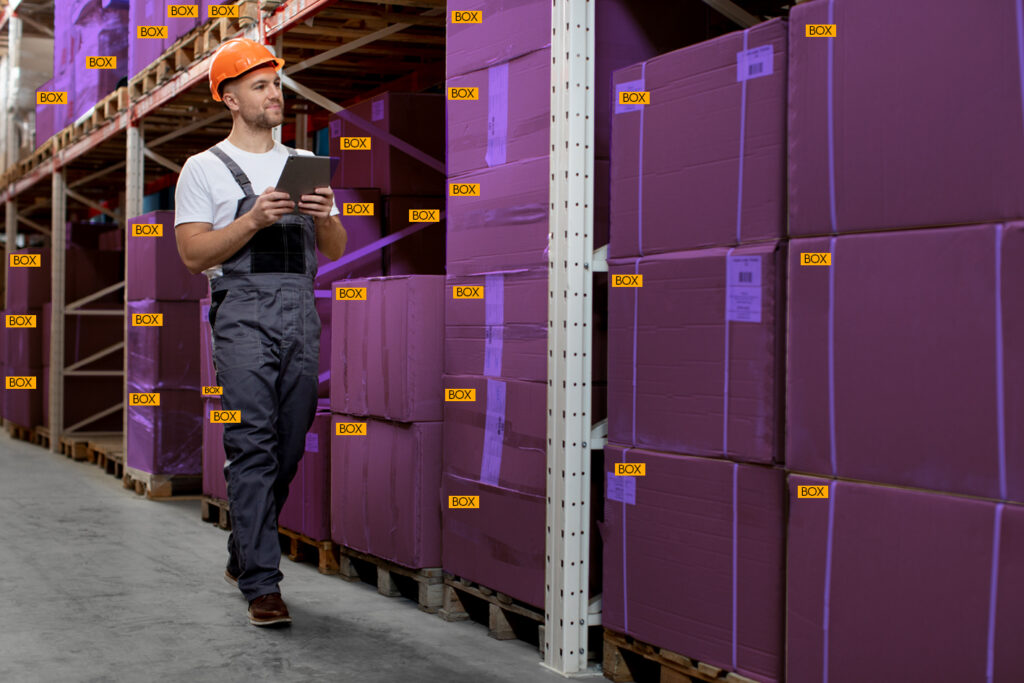
How Tackling Data Labeling Challenges in Logistics?
Tackling data labeling challenges in logistics is crucial to unlocking the full potential of AI-driven solutions. The logistics industry deals with massive volumes of diverse and complex data, from package tracking details to traffic patterns and warehouse inventory. Ensuring the accuracy and consistency of labeled data across such varied datasets can be daunting. Challenges such as managing large-scale labeling projects, maintaining data quality, and handling edge cases like mislabeled or ambiguous data points require robust strategies. Leveraging advanced labeling tools, outsourcing to specialized data labeling providers, and incorporating active learning techniques can help address these issues. By overcoming these challenges, companies can ensure that their AI systems are trained on reliable and high-quality datasets, enabling smarter, more efficient logistics operations.
Driving Sustainability in Logistics with AI
Sustainability is a top priority in modern logistics, and AI plays a pivotal role in achieving greener supply chains. Accurate data labeling enables AI systems to monitor carbon emissions, optimize energy usage, and minimize waste. Labeling vehicle telematics data enables AI models to suggest fuel-efficient routes and help lower emissions. Similarly, labeled data on material usage enables predictive analysis for reducing packaging waste. By integrating sustainability-focused metrics into labeled datasets, AI can help logistics companies meet environmental goals while maintaining profitability.
AI Applications in Logistics Enabled by Data Labeling
AI is transforming the logistics industry, enabling smarter, faster, and more cost-efficient operations. Key applications include route optimization, where AI analyzes real-time traffic data, delivery schedules, and weather conditions to recommend the most efficient paths. This minimizes fuel consumption and guarantees on-time deliveries. In predictive analytics, AI uses historical data to forecast demand, optimize inventory levels, and identify potential bottlenecks, allowing businesses to make proactive decisions.
AI also excels in real-time freight monitoring, providing live updates on shipment locations and conditions. With labeled datasets, AI models can identify patterns in traffic congestion, warehouse delays, or package dimensions, streamlining delivery operations. In warehouses, AI leverages labeled images and videos to train robots for inventory tracking, sorting, and navigating complex layouts. This improves operational accuracy, minimizes human errors, and accelerates order fulfillment processes, ultimately enhancing customer satisfaction. Let’s explore some key applications of AI in logistics and examine the critical role of data labeling in enabling these advancements.
Route Optimization
AI recommends optimal routes based on real-time traffic, weather data, and delivery schedules. Data labeling ensures that these models are trained with labeling maps and geospatial data, enabling accurate path predictions and reduced transit times.
Warehouse Automation
AI-driven robots transform warehouse operations from sorting and picking to inventory tracking. Data labeling enables robots to recognize objects and navigate complex layouts by providing labeled images of packages, shelves, and pathways.
Demand Forecasting
AI models analyze historical sales data and market trends to predict inventory needs accurately. Labeled datasets are crucial in training these models to identify patterns and forecast demand effectively, minimizing overstocking and understocking.
Autonomous Delivery Systems
AI drives drones and autonomous vehicles, enabling quicker and more efficient deliveries. Additionally, data labeling plays a crucial role by training these systems on labeled datasets, including roads, obstacles, and package handling scenarios. As a result, this ensures safe and reliable operations.
Sustainability and Emission Reduction
Accurately labeled data on energy usage and vehicle performance empowers AI to optimize fuel efficiency and lower carbon emissions, fostering more sustainable supply chains.
A Brief End
In conclusion, AI is redefining logistics by enabling smarter, more efficient, and sustainable supply chains. Furthermore, data labeling is pivotal in this transformation, providing the foundation for accurate and effective AI solutions. By powering advancements like route optimization, warehouse automation, and sustainability initiatives, data labeling ensures that AI models can address the challenges of modern logistics. As the industry progresses, prioritizing quality data labeling will, therefore, be key to unlocking AI’s full potential and driving future innovation in logistics.