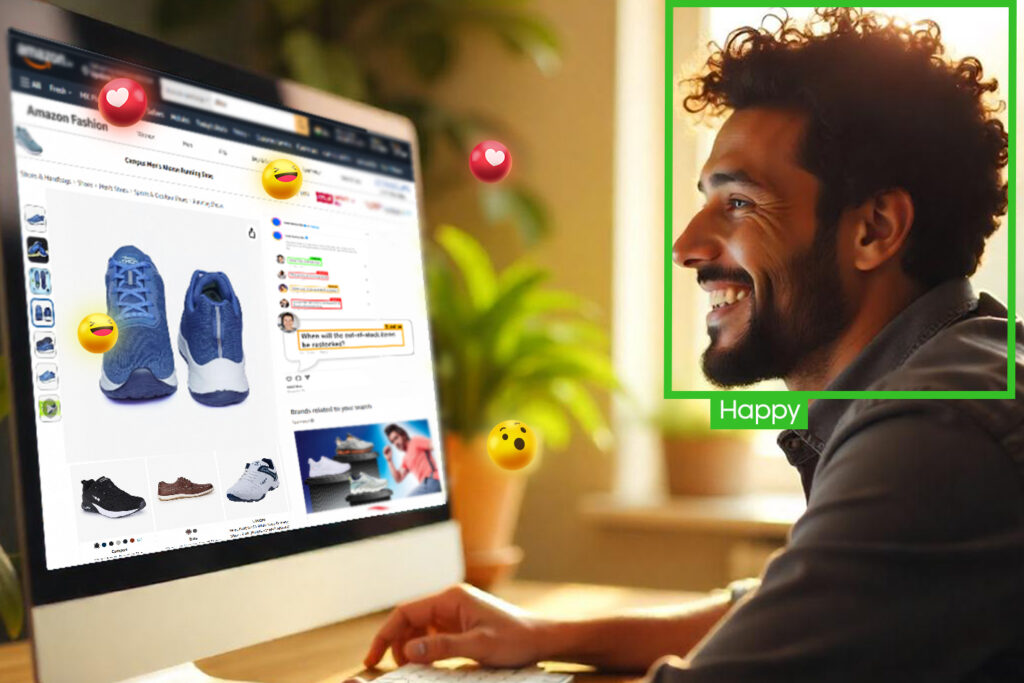
Social media has become a dynamic platform where millions of users share their thoughts, experiences, and opinions daily. Social media platforms produce vast amounts of unstructured data every second. From tweets and posts to comments and reviews, the digital footprint left by users holds invaluable insights into their opinions, preferences, and sentiments. Sentiment analysis, a subset of natural language processing (NLP), enables businesses and organizations to interpret this data and gauge public sentiment toward a brand, product, or topic. Sentiment analysis, driven by artificial intelligence, is one of the most popular methods to achieve this. However, despite the advancements in AI technologies, human attribution continues to play a critical role in ensuring the accuracy and reliability of sentiment analysis. However, while artificial intelligence can process vast amounts of data with unparalleled speed, it cannot completely replace the nuances brought by human attribution. This is where data labeling plays a pivotal role in ensuring the accuracy and reliability of sentiment analysis.
Dive deeper into how data labeling transforms sentiment analysis, revealing valuable insights and enhancing understanding in the digital era.
Why is Human Attribution Essential?
While AI can process data at unprecedented speeds, the need for human attribution in sentiment analysis cannot be overstated. Humans bring contextual understanding, emotional intelligence, and cultural knowledge qualities essential for interpreting nuanced social media content.
For example, a human analyst can identify the difference between genuine praise and sarcastic flattery, ensuring that sentiment classifications align with real-world perceptions. Human input is also invaluable during model training, where labelers help refine algorithms by validating and correcting AI-generated outputs.
The Role of AI in Social Media Sentiment Analysis
AI-powered sentiment analysis involves the use of natural language processing (NLP) algorithms to interpret and classify text into categories such as positive, negative, or neutral sentiments. The process can also identify emotions, sarcasm, and trends in social media conversations.
AI excels at handling massive datasets and performing real-time analysis, which is particularly beneficial for brands monitoring customer feedback or public opinion during events. For example, algorithms can scan millions of tweets, posts, and comments to reveal how people feel about a product launch or a political campaign.
Despite these capabilities, AI alone often struggles with nuanced language and cultural context. Understanding idioms, slang, and emojis in social media requires contextual knowledge that AI lacks. However, while artificial intelligence can process vast amounts of data with unparalleled speed, it cannot completely replace the nuances brought by human attribution. This is where data labeling plays a pivotal role in ensuring the accuracy and reliability of sentiment analysis.
The Role of Data Labeling
Data labeling is foundational to effective sentiment analysis. By labeling datasets with human-verified sentiment tags, AI models learn to associate specific words, phrases, and patterns with their respective sentiments.
Human labelers play a crucial role in identifying subtle cues, correcting biases, and ensuring that datasets reflect the diversity of real-world language. For instance, when dealing with sarcastic comments or region-specific slang, human labelers can provide labeling that clarifies intent. This labeled data improves the accuracy of AI models by enabling them to recognize complex language structures and emotional undertones.
Moreover, consistent labeling practices ensure that the training data remains robust and representative, allowing AI models to generalize better across different datasets. In essence, data labeling bridges the gap between raw, unstructured data and meaningful AI insights. For sentiment analysis to be effective, high-quality labeled datasets are essential. Data labeling ensures that the AI model learns to:
Distinguish Between Sentiments in Complex Scenarios:
- Social media content often includes subtle nuances that are hard to interpret, such as sarcasm, emojis, or double meanings. Data labeling helps AI understand these subtleties by providing clear examples and labelings.
Identify Patterns in User Behavior and Preferences:
- Data labelers tag content with relevant categories, allowing the AI model to recognize recurring themes and behavioral trends across vast datasets. This enables a deeper understanding of audience preferences and motivations.
Adapting to Changing Language Trends and Cultural Dynamics:
- Language on social media evolves rapidly, with new slang, abbreviations, and cultural references emerging frequently. Continuous data labeling keeps the AI updated, ensuring it remains relevant and accurate in its sentiment assessments.
Address Bias and Enhance Model Fairness:
- Humanlabelers can identify and correct biases in the training data, ensuring the AI delivers balanced and equitable sentiment analysis results.
Support Multilingual Sentiment Analysis:
- By labeling data across multiple languages, labeling enables AI to cater to diverse global audiences, making sentiment analysis more inclusive and comprehensive.
Bridging Recognition Models and Sentiment Analysis
Recognition technologies, such as face detection, gender classification, and pose estimation, share parallels with sentiment analysis in their reliance on precise data labeling. The methodologies used to label human features and gestures in images and videos can also enhance sentiment analysis.
For instance:
- Body Detection and Counting: Labeling human presence in images helps track audience participation in events, offering insights into public sentiment.
- Facial Recognition and Attribution: Detailed labeling of facial expressions and attributes can align with sentiment detection, enhancing emotional intelligence in AI models.
- Pose Estimation and Tracking: Understanding gestures can provide non-verbal cues to complement textual analysis, offering a holistic view of user sentiment.
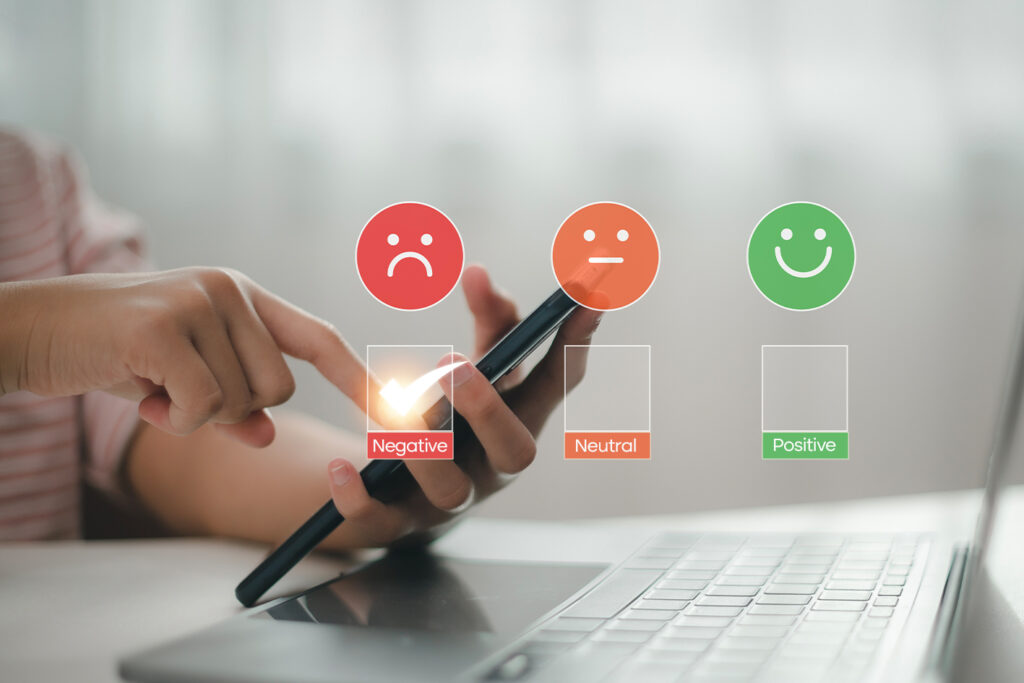
Conclusion
Sentiment analysis offers a powerful lens for interpreting vast and varied data on social media. While AI has significantly advanced the field, its limitations in understanding context, cultural nuances, and linguistic subtleties underscore the importance of human expertise. Data labeling and human attribution are pivotal in refining AI systems, ensuring accuracy, and mitigating biases.
By fostering a seamless collaboration between AI and human expertise, sentiment analysis tools become more precise and insightful. This synergy amplifies the potential of AI-driven social media sentiment analysis, enabling accurate interpretation of complex emotions and nuanced expressions. Data labeling and human attribution simplify social media, enabling deeper understanding.