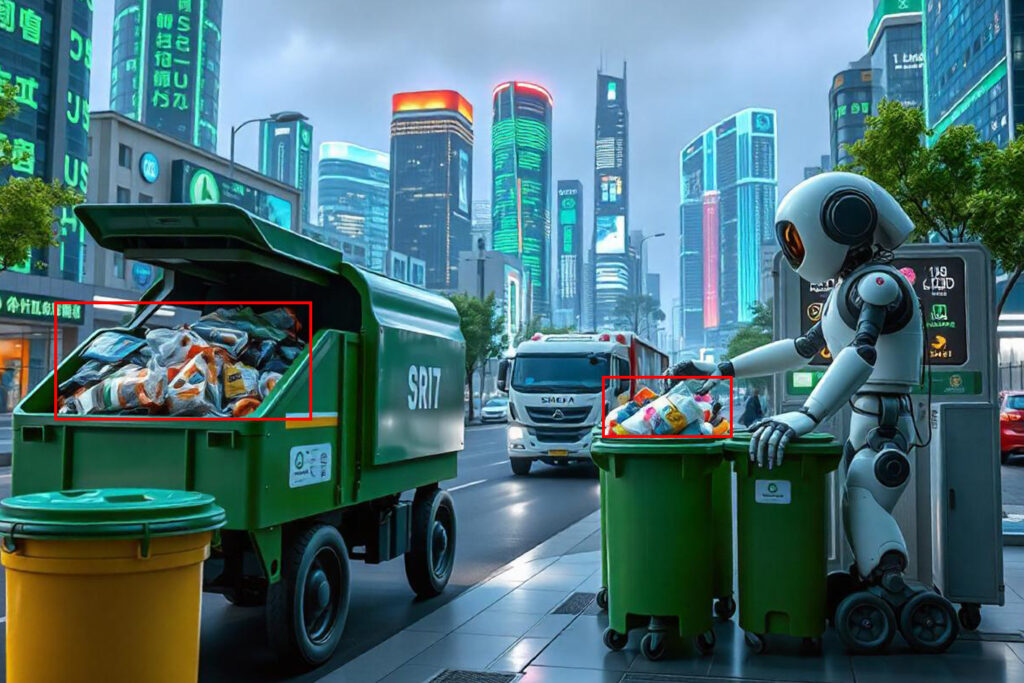
Efficient waste management has become a cornerstone of sustainable urban development, especially as cities face increasing waste volumes and environmental concerns. Rapid urbanization, growing populations, and changing consumption patterns have increased the strain on traditional waste collection systems. These systems, which operate on fixed schedules and routes, often result in overflowing bins, underutilized trucks, and excessive fuel consumption, leading to logistical inefficiencies and environmental hazards. Unmanaged waste leads to pollution, health risks, and greenhouse gas emissions, making efficient waste management vital globally.
Integrating Artificial Intelligence into waste collection offers a groundbreaking solution by optimizing routes and improving resource utilization. AI creates dynamic schedules tailored to actual needs by leveraging real-time data from IoT-enabled bins, GPS trackers, and traffic monitors. AI enhances waste collection by analyzing bin levels, traffic, and weather to minimize trips, reduce costs, and decrease carbon emissions. Additionally, advanced models analyze historical data to forecast waste patterns, allowing for more effective planning and resource allocation in sustainable waste management.
The Role of AI in Waste Collection
AI-driven waste management systems are transforming how cities handle waste collection, making the process smarter, faster, and more efficient. IoT-enabled smart bins with sensors monitor fill levels, waste composition, and usage frequency. These sensors collect real-time data and transmit it to centralized systems powered by artificial intelligence. Advanced AI algorithms analyze data to identify patterns, enabling waste collection to be customized based on area needs instead of fixed schedules.
For instance, if certain bins fill up faster than others due to high foot traffic in a commercial area, AI can prioritize those bins for immediate collection, preventing overflow and littering. Conversely, bins in low-usage areas are serviced only when necessary, eliminating unnecessary trips and conserving fuel. AI goes beyond bin data by factoring in live traffic, road closures, weather forecasts, and special events that impact waste generation and collection efficiency.
This dynamic and data-driven approach enables waste trucks to follow the most optimal routes, ensuring they spend less time on the road and more time efficiently collecting waste. AI also helps reduce wear and tear on vehicles, lowering maintenance costs and extending their lifespan. By combining real-time data with predictive analytics, these systems can anticipate waste collection needs, optimize fleet operations, and allocate resources more effectively.
Overall, AI’s role in waste collection goes beyond just route optimization; it creates a smarter, more efficient, and environmentally friendly system that improves urban cleanliness and supports long-term sustainability goals.
How Data Labeling?
The effectiveness of AI in waste collection heavily relies on accurate data labeling, which serves as the foundation for training AI models. High-quality labeled data ensures that AI systems can process and interpret real-world information precisely, allowing them to make decisions based on reliable insights. In the context of waste management, AI models rely on annotated datasets to differentiate various types of waste. For example, images captured by cameras in smart bins need to be labeled with the correct categories—plastic, organic, metal, paper, or hazardous materials. These labels help AI classify waste, predict bin fill levels, identify nearing capacity, and recommend recycling or disposal strategies.
Moreover, data labeling is essential in training machine learning models that manage traffic conditions and optimize waste collection routes. Traffic patterns, road closures, and congestion levels must be accurately labeled in historical data for AI models to make informed decisions on the best collection routes. This ensures that waste collection trucks avoid high-traffic areas and reduce fuel consumption, ultimately leading to lower operational costs and reduced environmental impact.
Another critical aspect of data labeling is its role in enhancing the accuracy of AI predictions. By continually labeling new datasets, AI models can learn from past patterns and refine their future predictions. For example, data labeled with seasonal variations in waste generation can help AI systems anticipate spikes in waste volumes during holidays or special events. This ensures that additional resources are allocated accordingly.
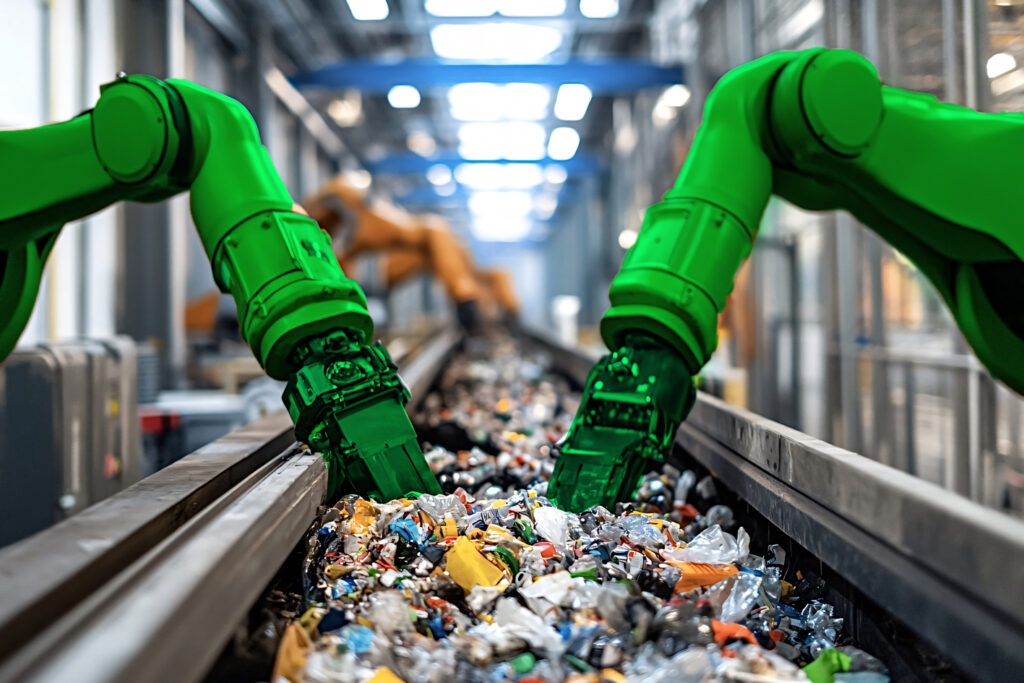
Benefits of AI and Data Labeling in Waste Management
AI-powered route optimization brings a range of benefits to urban waste management, enhancing both operational efficiency and sustainability:
- Reduced Environmental Impact:
AI optimizes travel routes and minimizes vehicle idling times. This reduces fuel consumption and lowers greenhouse gas emissions, contributing to cleaner air and a greener environment.
- Cost Savings:
AI-driven route planning reduces operational costs by cutting fuel expenses, lowering vehicle wear and tear, and decreasing maintenance needs, making waste management more affordable and efficient.
- Improved Efficiency:
AI enables timely waste pickups, preventing overflowing bins and maintaining a cleaner public environment. This optimized scheduling enhances overall service delivery for urban areas.
- Enhanced Recycling Efforts:
With accurate data labeling, AI can effectively categorize different types of waste, improving sorting processes. This process efficiently separates and processes recyclable materials, boosting recycling rates and supporting sustainable waste management practices.
Let’s Conclude
In conclusion, Data labeling plays a crucial role in AI-driven waste collection. It provides the accuracy and reliability needed to develop effective AI solutions. Data labeling drives smarter, sustainable waste management through accurate categorization, trend prediction, and optimized collection routes. High-quality labeled data is essential for AI to accurately sort waste, avoiding inefficiencies in recycling and disposal. Accurately categorizing waste ensures proper recycling of materials and safe handling of hazardous waste. AI, IoT, and data labeling collaborate to create a sustainable waste management system. This ensures cleaner cities and supports global sustainability goals, enabling precise, efficient, and responsible waste handling.