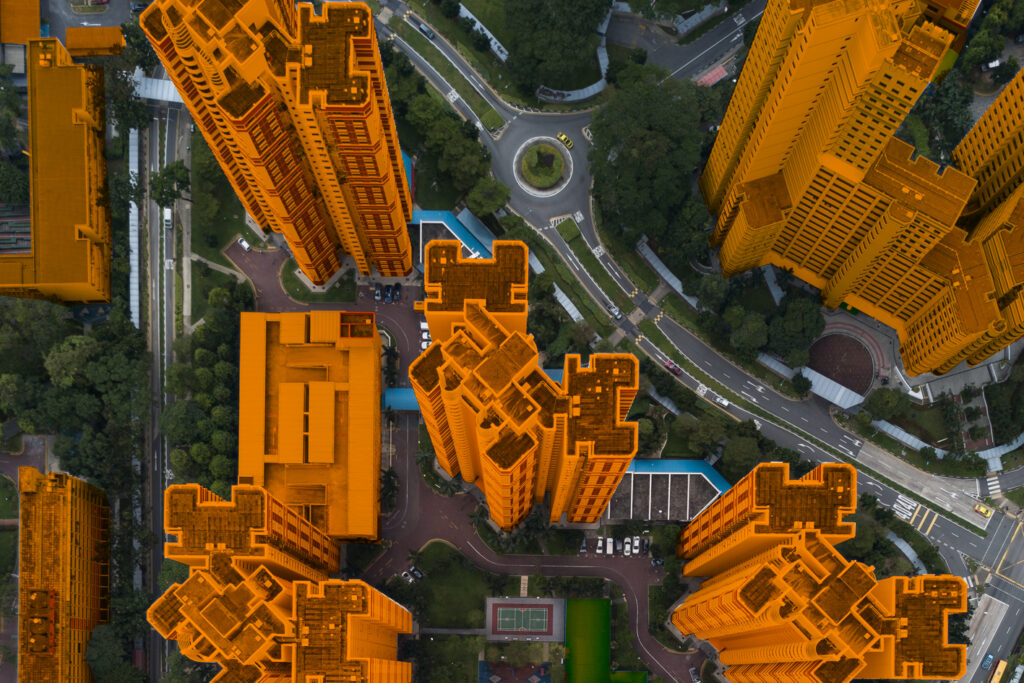
Urban planning is being transformed by advancements in artificial intelligence and geospatial technology. These advancements enable cities to tackle modern challenges with precision and efficiency, from managing rapid urbanization to addressing environmental concerns. Data labeling is at the heart of these innovations, a critical process that equips AI models to interpret and analyze complex geospatial data accurately.
Data labeling turns raw data into insights, driving smarter infrastructure, optimized resources, and better disaster management. AI-powered geospatial analysis also supports sustainable urban growth by identifying areas for conservation and helping cities reduce their environmental footprint. In this blog, we explore the transformative potential of AI and data labeling in urban planning. This powerful combination revolutionizes how cities are designed and managed, paving the way for smarter, greener, and more resilient urban environments. Let’s dive into more details.
The Importance of Geospatial Data in Urban Planning
Geospatial data comes from various sources, including satellite imagery, drones, and Geographical Information Systems (GIS). These data sources provide a detailed overview of urban environments, highlighting aspects such as land use, transportation networks, and natural resources. However, for AI to interpret this information effectively, precise labeling is essential. Data labeling identifies key features like roads, buildings, green spaces, and population density, enabling AI to analyze and forecast urban trends. These insights are invaluable for urban planners tasked with managing infrastructure, zoning, and sustainable development.
Data labeling is vital for transforming raw geospatial data into meaningful insights. It empowers AI systems to analyze and interpret complex datasets, enabling smarter decision-making across various domains. Let’s have a simple look at it.
Data Labeling Applications
Infrastructure Planning
Labeled geospatial data identifies ideal locations for roads, bridges, and public buildings, ensuring efficient resource use and reducing errors. It facilitates sustainable urban growth by forecasting long-term infrastructure requirements.
Traffic Management
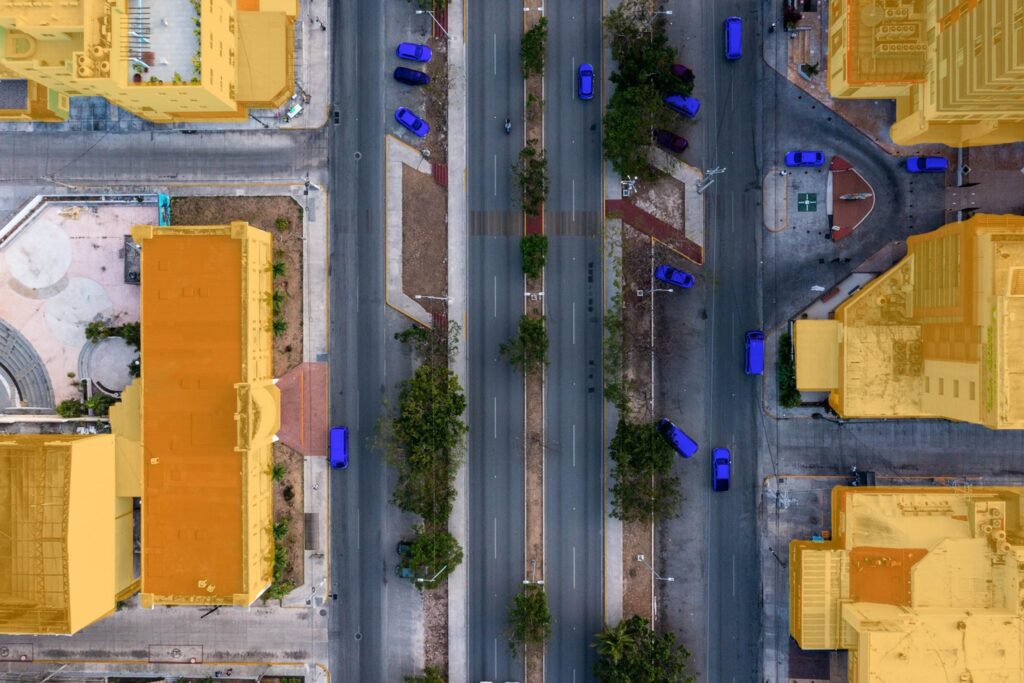
Labeled maps enable AI to analyze traffic flow, reduce congestion, and design efficient transport systems. Insights help optimize road networks, minimize emissions, and improve public commuting options.
Land Use Management
Labeled data categorizes land into zones like residential, commercial, or industrial. This enables effective resource allocation, balanced urban development, and future-ready city planning.
Disaster Management
Labeled data helps AI identify hazard zones, evacuation routes, and disaster-prone areas. It supports proactive planning and rapid response strategies, minimizing risks and saving lives.
Environmental Monitoring
Data labeling highlights green spaces, water bodies, and pollution levels. AI leverages this to promote sustainability, restore ecosystems, and create eco-friendly urban designs.
Military Operations
Geospatial data labeling enhances military operations by improving surveillance, mission planning, border security, and disaster relief. It supports autonomous systems, asset tracking, and cybersecurity for critical infrastructure.
Smart City Development
Data labeling supports AI in designing smart infrastructure, from intelligent traffic lights to IoT-based waste management systems.
Challenges in Geospatial Data Labeling
Geospatial data labeling involves significant challenges, such as handling vast datasets generated from sources like satellite imagery and drone technology. Accuracy is crucial, as even minor errors can affect AI model outcomes. The process demands skilled labelers to interpret complex patterns and deal with issues like varying resolutions, noise, and occlusions. Ensuring consistency across labeling and addressing these complexities requires advanced tools and expertise for reliable and precise results.
High Complexity
Urban landscapes include intricate features like buildings, roads, and waterways. Labeling these elements demands advanced techniques and expert precision, making the process time-consuming and complex. Variability in layouts across regions adds to the challenge.
Data Volume
Geospatial datasets from satellites, drones, and GIS are massive, requiring robust infrastructure for processing and storage. Managing this scale can overwhelm resources, particularly for organizations with limited capacity.
Accuracy Demands
Accurate labeling is crucial for reliable AI predictions, as errors can impact urban planning and disaster management. Precision often requires skilled labelers combined with AI-assisted tools.
Dynamic Environments
This dynamic nature demands real-time monitoring and adaptive labeling processes to ensure AI models stay relevant and accurate in their analyses.
Cost and Resource Constraints
Labeling large datasets is expensive, requiring skilled labor and advanced tools. This poses challenges for organizations with limited resources, making it hard to balance costs and quality.
The Future of Urban Planning with AI and Data Labeling
AI and data labeling are transforming urban planning into a predictive and proactive process. Machine learning models can forecast challenges like population growth, traffic congestion, and climate change, enabling planners to address issues before they escalate.
Innovations such as augmented reality, 3D geospatial mapping, and digital twins are enhancing planning with immersive visualization tools and real-time simulations. These technologies improve resource allocation, disaster preparedness, and public engagement, empowering cities to adapt and thrive.
High-quality data labeling remains the backbone of these advancements, ensuring AI delivers accurate insights to build smarter, more resilient urban spaces.
Let’s Conclude
Geospatial data labeling is transforming the way cities are planned and managed, making it a cornerstone of modern urban development. By enabling AI to extract actionable insights from complex datasets, it empowers urban planners to address critical challenges like traffic congestion, infrastructure inefficiencies, and environmental degradation. Labeled data helps ensure that resources are allocated wisely, infrastructure is designed with precision, and urban spaces are optimized for both functionality and sustainability.
Geospatial labeling enhances disaster management by identifying risk zones and planning evacuation routes, improving preparedness for unforeseen events. Beyond immediate needs, it drives long-term strategies for smarter, greener, and more connected cities. As urban areas evolve, labeling data remains vital for building resilient and adaptive urban environments.